

The Probability Calculation step defines how these probabilities are calculated.įor example, let's say we’re interested in two different probabilities: Probability CalculationĪs mentioned before, coherence metrics use probabilities drawn from the textual corpus. This fact highlights an important point of the topic coherence measures: it depends not only on the topic itself but also on the dataset used as reference.Īgain, by using this technique, we’re saying that our coherence score will be based on the relation between a single word and the rest of the words in our topic. It uses statistics and probabilities drawn from the reference corpus, especially focused on the word’s context, to give a coherence score to a topic. What a Topic Coherence Metric assesses is how well a topic is ‘supported’ by a text set (called reference corpus). For example, a set of arguments is coherent if they confirm each other. Usually, when we talk about coherence, we talk about a cooperation characteristic. The first key point to understand how these metrics work is to focus on the word ‘Coherence’. They try to represent the ‘quality human perception’ about topics in a unique, objective, and easy-to-evaluate number. This is the problem Topic Coherence Measures try to solve.

It also requires prior knowledge about the dataset’s field and may require specialist opinions. Unfortunately, this task can be very time-consuming and impracticable to very large datasets with thousands of topics. So, just blindly following the inherent math behind topic model algorithms can lead us to misleading and meaningless topics.īecause of this, topics’ assessment was usually complemented by qualitative human evaluations, such as reading the most important words in each topic and seeing the topics related to each document. When we’re looking for data understanding, the topics created are meant to be human-friendly. Sometimes, we don't need that the topics created to follow some interpretable logic, and we just want to, for example, reduce the data dimensionality to another machine learning process. (Probably a bad topic)įrom a human point of view, the first topic sounds more coherent than the second but, for the algorithm, they are probably equally correct. However, mathematically optimal topics are not necessarily ‘good’ from a human point of view.įor example, a topic modeling algorithm can find the following topics: As described before, topic modeling algorithms rely on mathematics and statistics. Evaluating TopicsĬomputers are not humans. The words with the highest values can be considered as the true participants of a topic.įollowing this logic, our previous example will be something like this: So, a Topic Modeling Algorithm is a mathematical/statistical model used to infer what are the topics that better represent the data.įor simplicity, a topic can be described as a collection of words, like and, but in practice, what an algorithm does is assign each word in our vocabulary a ‘participation’ value in a given topic. A topic is composed of a collection of words.A text (document) is composed of several topics.It aims to explain a textual dataset by decomposing it into two distributions: topics and words.
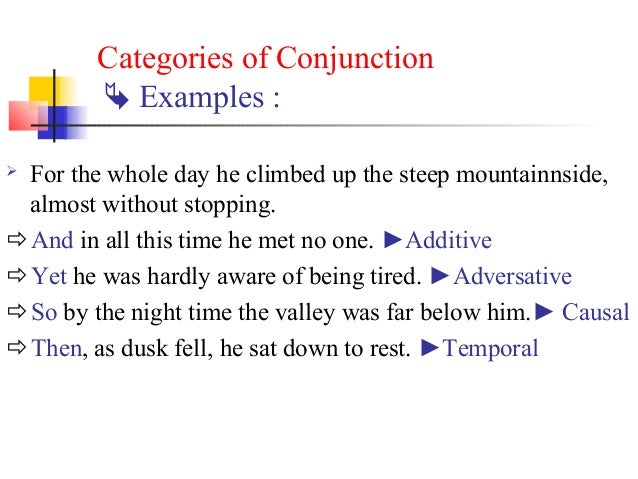
Topic Modeling is one of the most important NLP fields. How Topic Coherence Works - Segmentation - Probability Calculation - Confirmation Measure - Aggregation - Putting everything together IV.
